AI Revolution: Navigating the Risks and Security Challenges in the Intelligent Era
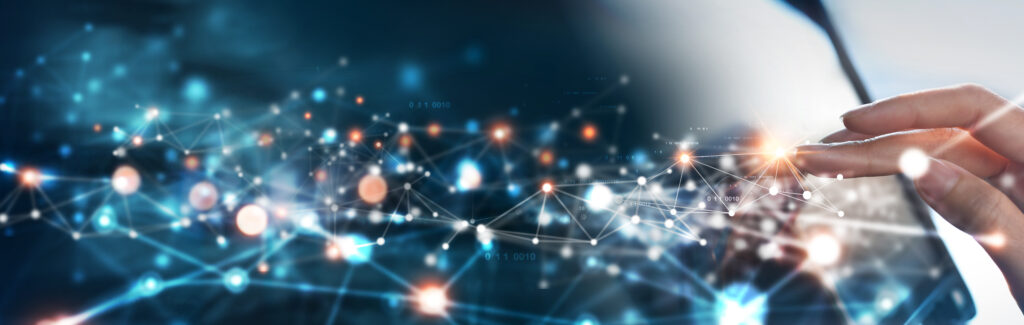
AI is rapidly changing how we do business and develop tech. It’s powerful, but it brings new security risks we can’t ignore. This post breaks down the complexities of AI systems, the threats they face, and how we can bake security into AI from the ground up.
AI has seen a rapid advancement in terms of the technology and the abilities that it brings. Over the past two years generative AI has progressed at such a rate that most large corporations have struggled to keep up. Incorporating AI in their business processes, both internally and externally, has been on the rise, and we are seeing this trend increase every day.
With the boom that came from ChatGPT, we have also seen new attacks targeting AI technology. From prompt injections to model poisoning, we are seeing new threats and vulnerabilities that exist only for AI services and applications. Although these attacks are new in their nature, the underlying security concepts we have today are still relevant, where protecting data and managing access are paramount.
This change has brought with it a need for a shift on governance and compliance, as traditional systems a largely unsuited for AI governance. There are multiple factors that need to be addressed, such as increased scale and complexity, reliance on existing models, and management of sensitive and personal data.
Complexity and Scale of AI Systems
Many AI systems are leveraging a large amount of data and processing capabilities. Other than the increased costs of storge and performance, management of such quantities of data – and often sensitive data – has not been a concern for traditional systems. The ability to serve, monitor, and store in such large volumes must be addressed while still maintaining the same level of data sovereignty and compliance with regulations that most businesses are obliged to follow. This can lead to inefficiencies, downtime, and even vulnerabilities that expose the data or models themselves.
The complexity of AI systems is further enhanced by the nature of retraining models and fine-tuning. To confidently maintain accuracy, datasets must increase and update to feed the everlasting development and growth of AI. Traditional systems generally work with static data that can be tested with proven knowable outcomes, whereas generated outputs from LLMs can adjust and evolve from one moment to the next.
Decisions and Explanations
AI has the capacity for decision-making, automating areas of systems that require governance and accountability. Due to the nature of AI’s decision-making process, where defining how outputs trace back from inputs is as complex as the system itself, explainability is replaced with efficiency and driven by results instead of understanding the process. Areas of governance such as ethical considerations and regulatory compliance require tools that can overcome this lack of transparency.
Shaping the fairness of an intelligent system also comes under fire, where unintentional bias can go undetected and presents opinion over fact when inadequately monitored and mitigated. This poses a substantial risk in ethical AI deployment, in a constantly evolving landscape of legal compliance across the globe.
Privacy and Security
Privacy concerns have been raised where AI systems have access to sensitive information in order to fulfil their requirements. Efforts to manage this data for anonymisation, consent, retention, and required protection is vastly increased and can become a struggle both for the resources required and the complexity of the systems, leading to possible breaches of data and non-compliance with regulations.
Cybersecurity has seen new types of attacks designed specifically for AI systems, manipulating outputs and exploiting vulnerabilities for various ends. Trust needs to be complete for any AI systems to be of value, therefore overcoming these threats is paramount and requires continuous vigilance. In these early stages of commercial AI systems, it is difficult to foresee the vectors of attack with such a large surface that is ever-growing. Reaction to discovered threats needs to be swift, with a heavy focus on asserting that weaknesses are removed, and systems are not compromised.
Building Security into AI
Fundamentally, AI systems are complex programs – but programs, nonetheless. Observations of security best practices has not changed here, using Zero Trust principles across organisations and development operations to ensure access controls are robust and data is stored safely and securely. At XAM, we follow these principles in our development to prevent unauthorised access to data and secrets using encryption, secure storage, and service principals to access only what is required. The same principles are used when developing AI systems, leveraging keyless authentication to services to prevent the need for API or service keys during development or at runtime.
Data storage, regardless of volume or type, can be secured using access controls, encryption and retention policies whilst still observing requirements to governance and compliance. Separation of environments, including access controls to data storage, ensures the security of systems across production and development throughout the software development lifecycle.
Authentication has always been a big ticket in application development, and AI is no different. In the current landscape, multi-factor authentication is a benchmark for securing user access – but that is not where authentication stops. Data access control in Azure allows us to define what data can be used to generate responses in AI applications, meaning that sensitive data or restricted documents can be filtered out of datasets for certain users – protecting your data while delivering a solution that implements a productive and secure application.
Building AI isn’t just about making smart systems; it’s about making them secure. As we push AI further, we need to stay on top of its unique security challenges. By focusing on solid data protection, tight access control, and ethical AI practices, we can build AI systems that are both powerful and trustworthy. The future of AI looks promising, but it’s on us to make sure it’s safe and responsible. Let’s build AI that not only boosts our capabilities but also keeps our data and values intact.
Interested in exploring these concepts with XAM? Book your discovery here!